Learning:Evolution
Mapping an analogy to understand adaptive change within and between generations
Learning and evolution are both processes of adaptive changeÂ
that are simultaneously similar and different from each other.
What can humans learn by comparing these processes?
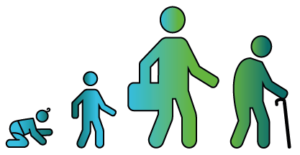
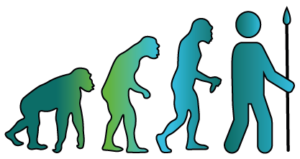
Learning is like evolution in that our knowledge, understanding and skills change over time, helping us adapt to our environments
Evolution is like learning in that species tend to change over time, becoming better adapted to their environments.
Of course, learning and evolution are different concepts!
How can we understand these similarities and differences?
The Learning:Evolution Analogy in Science
There is a long history and diverse range of traditions across disciplinary perspectives that have profitably explored the similarities and differences between learning and evolution.Â
However, despite this rich history of scientific thought, many scientists within any given tradition may never learn of or explore the merits and limits of this analogy.Â
Critically, the educational sciences represent a domain in which the learning:evolution analogy is particularly relevant, yet virtually unexplored.



Mapping the Learning:Evolution Analogy
The value of any analogy is in the careful and critical mapping of specific dimensions of similarity and difference.
Thus, to understand the Learning:Evolution analogy, we must look at the conceptual relations between specific dimensions in each process. There are many ways to do this (as we discuss in Hanisch & Eirdosh 2023, in press). Table 1, below, is just one possible example of mapping core concepts in evolutionary theory across domains of genetic, cognitive, and cultural evolution. Table 2 presents the influential model from Cognitive Load Theorist, John Sweller (see Sweller & Sweller 2006), looking at evolution and cognition as examples of natural information processing systems. Table 3 provides an alternative framing, focused instead on the concepts of agential and decentralized causes across canonical cases of learning and evolution.
Critically, the value of the analogy is not in describing different phenomena as being “the same”, but in identifying which abstracted concepts may be relevant across very diverse domains. There is not one “optimal” way of doing this, but rather, various formulations may have different value, and these diverse formulations may have particularly different functions in the context of scientific research versus education. The key in all cases is to have logical and justified reasons for clarifying a shared concept across differing domains.Â
Table 3. An analogy map describing concepts of agential and decentralized causation, as applied across domains of machine learning, human learning, and biological evolution (Eirdosh & Hanisch, in prep; see also The Triad).
The Learning:Evolution Analogy in Education
One important reason why the learning:evolution analogy has not been explored in education is perhaps related to the conceptual challenges of reasoning about the role of agency, in learning, and in evolution.Â
Intuitively, many people think of learning as a process driven by the agency, the goal-directed behaviors, of individuals or communities. A student either chooses or is required to learn something within the school curriculum.Â
In contrast, some within the field of evolution education emphasize that evolution is a process not driven by agency, but by the processes of natural selection.Â
In fact, in contrast to both of these popular conceptions, both learning and evolution often include processes that are partially influenced by the goal-directed behaviors of agents, and partially influenced by other (decentralized, non-agential) factors.Â
By using thinking tools developed within the Understanding Agency project, students can explore the often complex interplay between agential and decentralized causes within processes of learning, as well as evolution.Â
Other tools for Understanding Agency
Deepen your understanding of agency across diverse contexts through other resources in the collection.Â
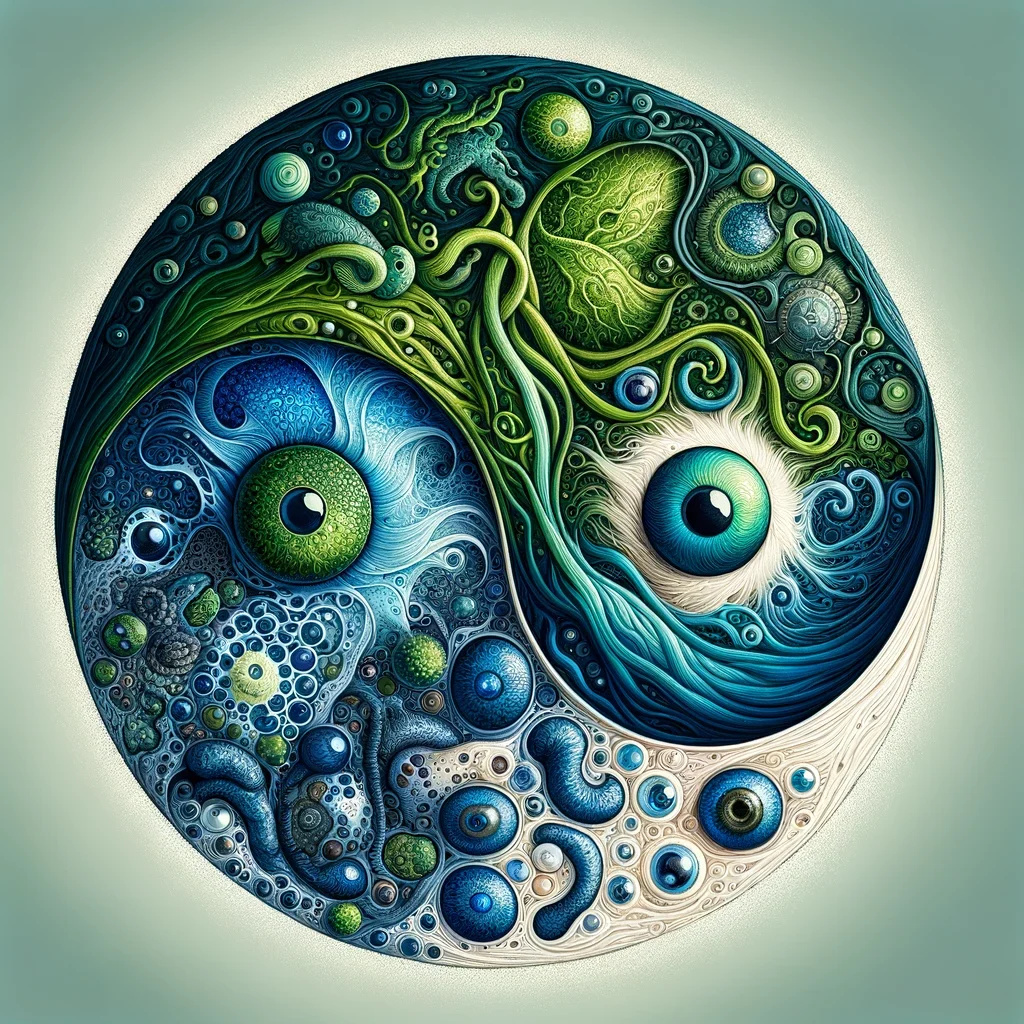
Understanding Agency
Tools for thinking about the origins, diversity, and flexibility of goal-directed behaviors
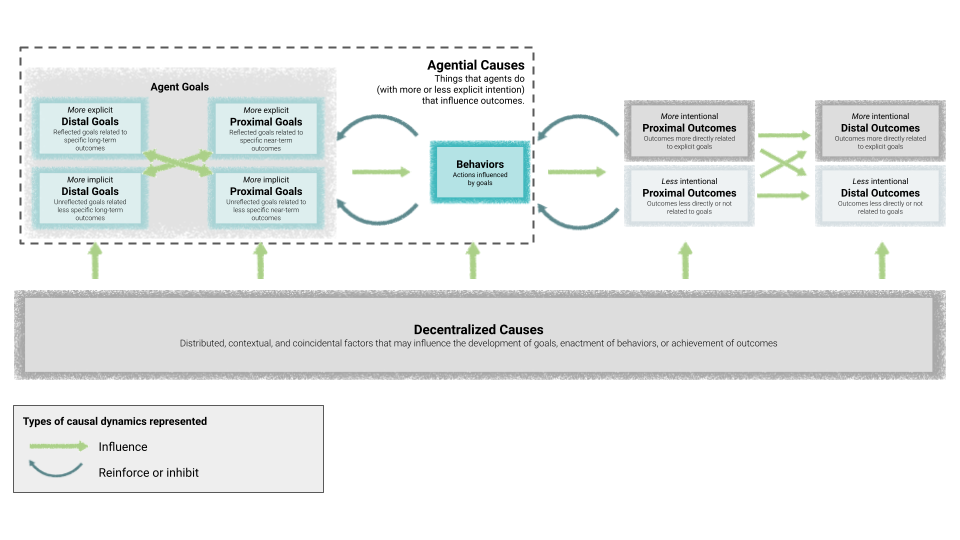
Goals, Behaviors, and Outcomes
How can we understand the causal relationships between goals, behaviors, and outcomes?
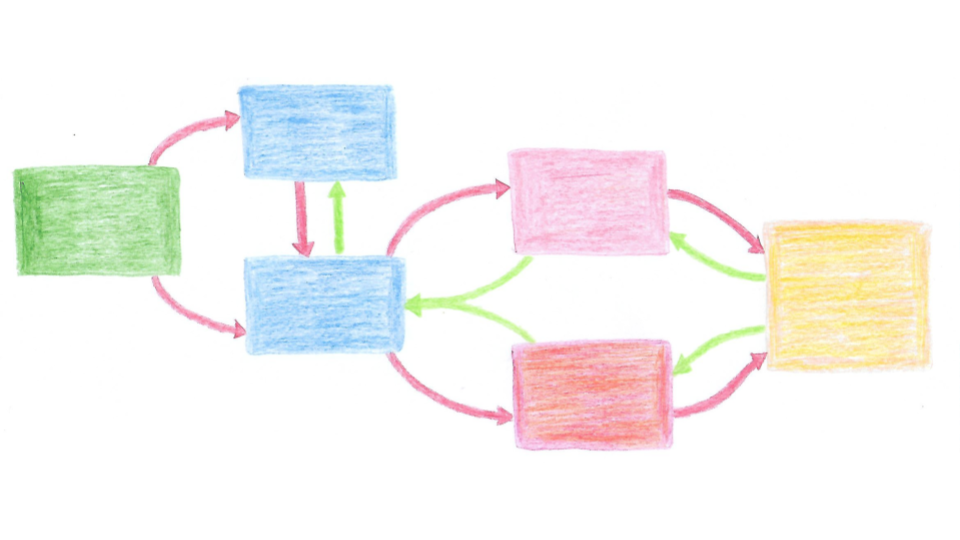
Agency in Evolution Education
A page for researchers interested in the concept of agency as it relates to learning theories of evolution, including natural selection in biological systems.
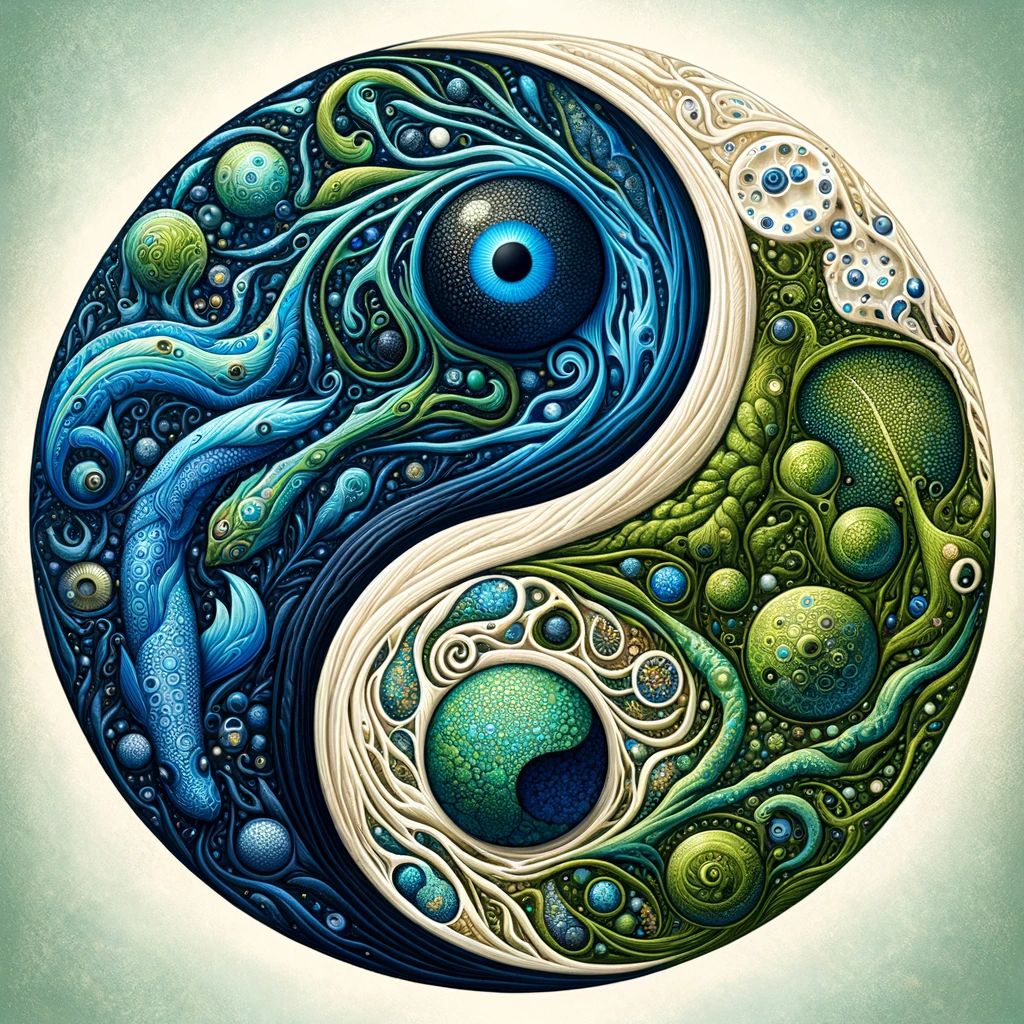
The Decentralized Self
The human brain is the seat of human agency, and yet this agency is caused by cellular agents unaware of our larger human goals.
References and further reading
Borgstede, M., & Eggert, F. (2021). The formal foundation of an evolutionary theory of reinforcement. Behavioural processes, 186, 104370. https://doi.org/10.1016/j.beproc.2021.104370
Hanisch, S. & Eirdosh, D. (2023). Teaching for the interdisciplinary understanding of evolutionary concepts.Chapter in du Crest et al. (2023). Evolutionary Thinking Across Disciplines.Problems and Perspectives in Generalized Darwinism.
Monestès, J.-L. (2015). A Functional Place for Language in Evolution. In R. D. Zettle, S. C. Hayes, D. Barnes-Holmes, & A. Biglan (Eds.), The Wiley Handbook of Contextual Behavioral Science (pp. 100–114). Wiley & Sons. https://doi.org/10.1002/9781118489857.ch7
Nardo, A. (2018). The evolutionary foundations of John Dewey’s concept of growth and its meaning for his educational theory. Zeitschrift für Pädagogik, 64(6), 852-870.
Nardo, A. (2021). Exploring a Vygotskian theory of education and its evolutionary foundations. Educational Theory, 71(3), 331-352. https://onlinelibrary.wiley.com/doi/epdf/10.1111/edth.12485
Popp, J. A. (2012). Evolution’s First Philosopher: John Dewey and the Continuity of Nature. SUNY Press.
Rahwan, I., Cebrian, M., Obradovich, N., Bongard, J., Bonnefon, J. F., Breazeal, C., … & Wellman, M. (2019). Machine behaviour. Nature, 568(7753), 477-486.
Richerson, P. J. (2019). An integrated Bayesian theory of phenotypic flexibility. Behavioural Processes, 161, 54-64.
Rosenbaum, D. A. (2014). It’s a Jungle in There: How Competition and Cooperation in the Brain Shape the Mind. Oxford University Press.
Skinner, B. F. (1953). Science and Human Behavior. New York, USA: Macmillan
Sweller, J., & Sweller, S. (2006). Natural information processing systems. Evolutionary Psychology, 4(1), 147470490600400135.
Wasserman, E. A. (2021). As if by design: How creative behaviors really evolve. Cambridge University Press.
Watson, R. A., & Szathmáry, E. (2016). How can evolution learn?. Trends in Ecology & Evolution, 31(2), 147-157.
Wilson, D.S., Hayes, S.C. (Eds.) (2018). Evolution and contextual behavioral science: An integrated framework for understanding, predicting, and influencing human behavior. New Harbinger Publications.